Hadoop 生态圈(二)- HDFS 操作详解
|字数总计:3.8k|阅读时长:15分钟|阅读量:
前言
部分内容摘自尚硅谷、黑马等培训资料。
1. HDFS概述
1.1 HDFS产生背景及定义
1.1.1 HDFS产生背景
随着数据量越来越大,在一个操作系统存不下所有的数据,那么就分配到更多的操作系统管理的磁盘中,但是不方便管理和维护,迫切需要一种系统来管理多台机器上的文件
,这就是分布式文件管理系统。HDFS只是分布式文件管理系统中的一种。
1.1.2 HDFS定义
HDFS(Hadoop Distributed File System)
,它是一个文件系统,用于存储文件,通过目录树来定位文件;其次,它是分布式的,由很多服务器联合起来实现其功能,集群中的服务器有各自的角色。
HDFS的使用场景:适合一次写入,多次读出的场景。
一个文件经过创建、写入和关闭之后就不需要改变。
HDFS 主要适合去做批量数据出来,相对于数据请求时的反应时间,HDFS 更倾向于保障吞吐量。
1.1.3 HDFS发展史
- Doug Cutting 在做 Lucene 的时候, 需要编写一个爬虫服务,这个爬虫写的并不顺利,遇到了一些问题, 诸如:如何存储大规模的数据,如何保证集群的可伸缩性,如何动态容错等。
- 2013 年,Google 发布了三篇论文,被称作为三驾马车,其中有一篇叫做 GFS。
- GFS 是描述了 Google 内部的一个叫做 GFS 的分布式大规模文件系统,具有强大的可伸缩性和容错性。
- Doug Cutting 后来根据 GFS 的论文,创造了一个新的文件系统,叫做 HDFS
1.2 HDFS优缺点
- 优点
高容错性
- 数据自动保存多个副本。它通过增加副本的形式,提高容错性。
- 某一个副本丢失后,它可以自动恢复。
适合处理大数据
- 数据规模:能够处理数据规模达到GB、TB甚至PB级别的数据。
- 文件规模:能够处理百万规模以上的文件数量,数量相当之大。
可构建在廉价机器上
,通过多副本机制,提高可靠性。
- 缺点
不适合处理低延时数据访问
,比如毫秒级的存储数据,是做不到的。
无法高效的对大量小文件进行存储
- 存储大量小文件的话,它会占用 NameNode 大量的内存来存储文件目录和块信息。这样是不可取的,因为 NameNode 的内存总是有限的。
- 小文件存储的寻址时间会超过读取时间,它违反了 HDFS 的设计目标。
不支持并发写入、文件随机修改
- 一个文件只能有一个写,不允许多个线程同时写。
- 仅支持数据 append,不支持文件的随机修改。
2. HDFS组成架构
- NameNode: Master,它是一个主管、管理者。
- 管理 HDFS 的名称空间;
- 配置副本策略;
- 管理数据块(Block)映射信息;
- 处理客户端读取请求;
- DataNode: Slave,NameNode赋值下达命令,DataNode执行实际的操作。
- Client: 客户端。
- 文件切分。文件上传 HDFS 的时候,Client 将文件切分成一个一个的Block,然后进行上传;
- 与 NameNode 交互,获取文件的位置信息;
- 与 DataNode 交互,读取或者写入数据;
- Client 提供一些命令来管理 HDFS,比如对 NameNode 格式化;
- Client 可以通过一些命令来访问 HDFS,比如对 HDFS 增删改查操作;
- SecondaryNameNode: 并非 NameNode 的热备,当 NameNode 挂掉的时候,它并不能马上替换 NameNode 并提供服务。
- 辅助 NameNode,分担其工作量,比如定期合并 Fsimage 和 Edits,并推送给 NameNode;
- 在紧急情况下,可辅助恢复 NameNode;
3. HDFS的重要特性
3.1 主从架构
HDFS 采用 master/slave 架构。一般一个 HDFS 集群是有一个 Namenode 和一定数目的 Datanode 组成。Namenode是HDFS主节点,Datanode是HDFS从节点
,两种角色各司其职,共同协调完成分布式的文件存储服务。
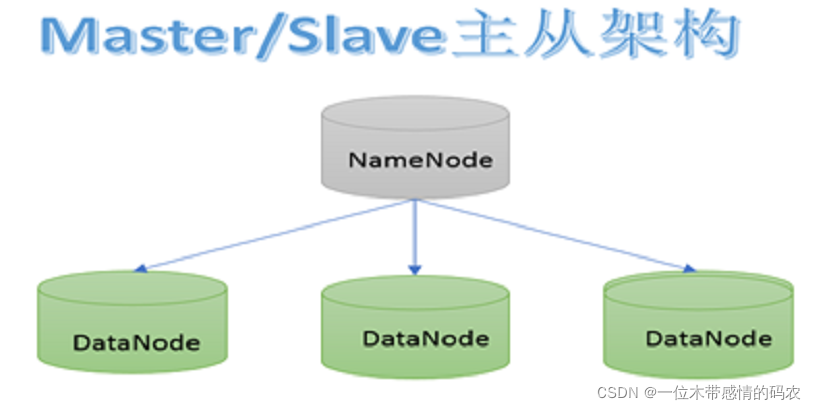
3.2 分块机制
HDFS 中的文件在物理上是分块存储(block)的,块的大小可以通过配置参数来规定,参数位于 hdfs-default.xml 中:dfs.blocksize。默认大小在 Hadoop2.x/3.x 是128M(134217728)
,1.x 版本中是 64M。
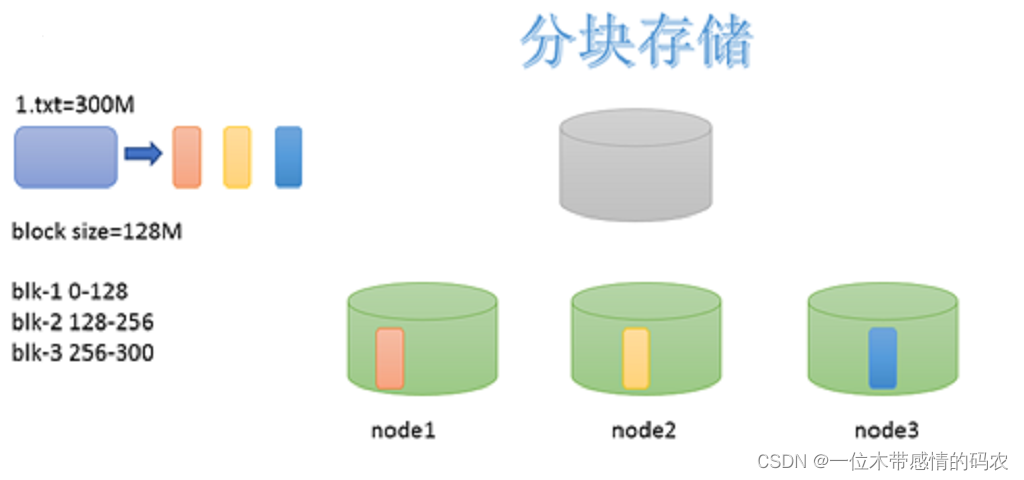
3.2.1 HDFS文件块大小设置
- HDFS 的块设置太小,会增加寻址时间,程序一直在找块的开始位置;
- 如果块设置的太大,从磁盘传输数据的时间会明显大于定位这个块开始位置所需的时间,导致程序在处理这块数据时,会非常慢。
总结:HDFS块的大小设置主要取决于磁盘传输速率。
3.3 副本机制
为了容错,文件的所有 block 都会有副本。每个文件的 block 大小(dfs.blocksize)和副本系数(dfs.replication)都是可配置的。应用程序可以指定某个文件的副本数目。副本系数可以在文件创建的时候指定,也可以在之后通过命令改变。
默认dfs.replication的值是3
,也就是会额外再复制 2 份,连同本身总共 3 份副本。
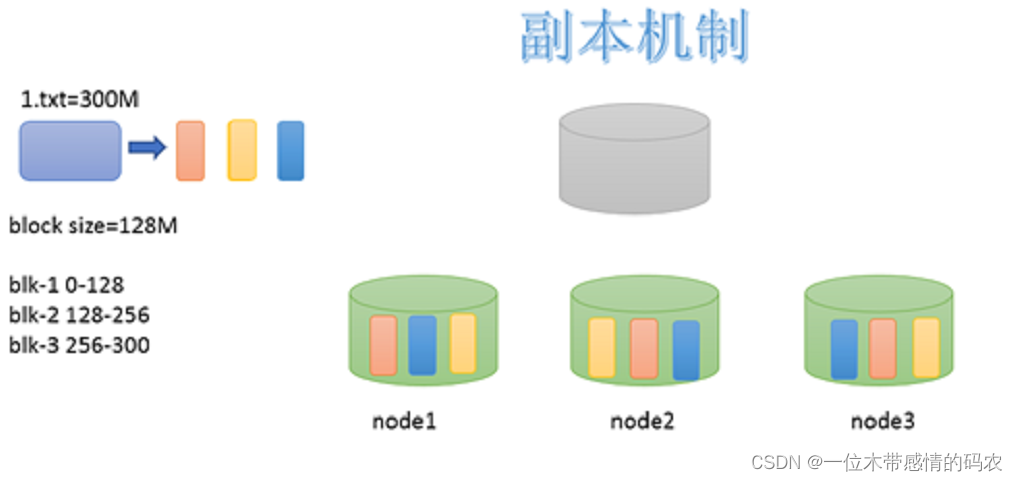
3.4 Namespace
HDFS 支持传统的层次型文件组织结构
。用户可以创建目录,然后将文件保存在这些目录里。文件系统名字空间的层次结构和大多数现有的文件系统类似:用户可以创建、删除、移动或重命名文件。
Namenode 负责维护文件系统的 namespace 名称空间,任何对文件系统名称空间或属性的修改都将被 Namenode 记录下来。
HDFS 会给客户端提供一个统一的抽象目录树
,客户端通过路径来访问文件,形如:hdfs://namenode:port/dir-a/dir-b/dir-c/file.data。
3.5 元数据管理
在 HDFS 中,Namenode 管理的元数据具有两种类型:
文件自身属性信息
- 文件名称、权限,修改时间,文件大小,复制因子,数据块大小。
文件块位置映射信息
- 记录文件块和 DataNode 之间的映射信息,即哪个块位于哪个节点上。
3.6 数据块存储
文件的各个 block 的具体存储管理由 DataNode 节点承担。每一个 block 都可以在多个 DataNode 上存储。
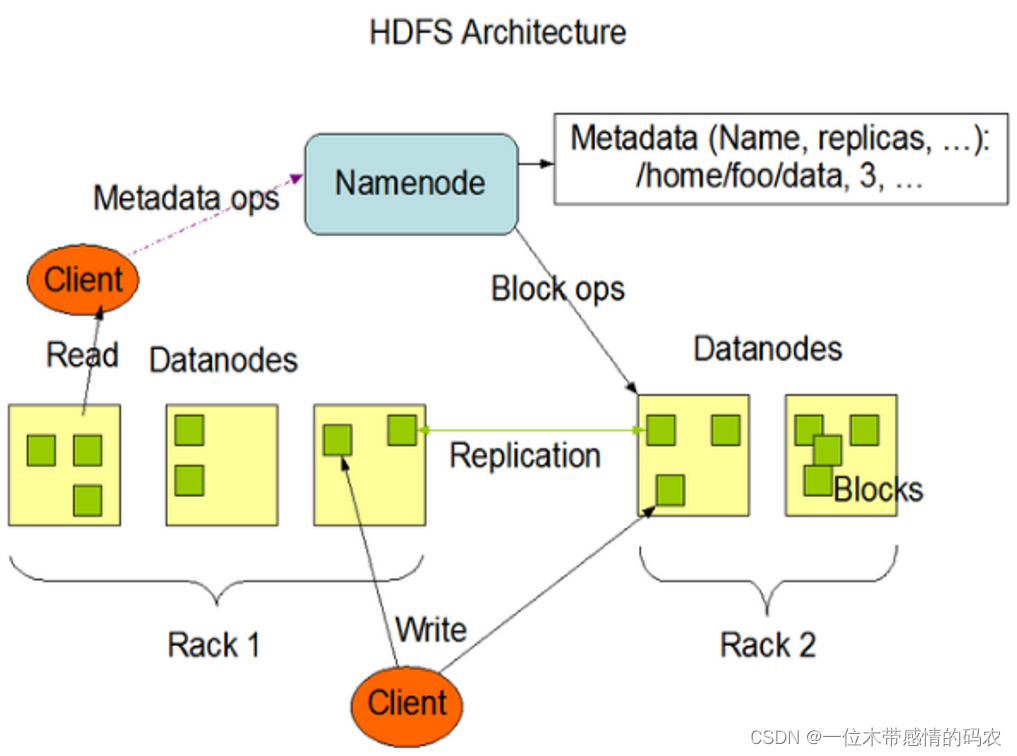
4. HDFS的Shell操作
4.1 基本语法
1
| hadoop fs 具体命令 OR hdfs dfs 具体命令
|
4.2 上传
-moveFromLocal
:从本地剪切
粘贴到 HDFS
1
| hadoop fs -moveFromLocal ./1.txt /test
|
-copyFromLocal
:从本地文件系统中拷贝
文件到 HDFS 路径去
1
| hadoop fs -copyFromLocal ./1.txt /test
|
-put
:等同于 copyFromLocal,生产环境更习惯用 put
1
| hadoop fs -put ./1.txt /test
|
-appendToFile
:追加一个文件到已经存在的文件末尾
1
| hadoop fs -appendToFile ./1.txt /test/1.txt
|
4.3 下载
-copyToLocal
:从 HDFS 拷贝
到本地
1
| hadoop fs -copyToLocal /test/1.txt /output
|
-get
:等同于 copyToLocal,生产环境更习惯用 get
1
| hadoop fs -get /test/1.txt /output
|
4.4 HDFS直接操作
1
| hadoop fs -cat /test/1.txt
|
-chgrp
、-chmod
、-chown
:Linux 文件系统中的用法一样,修改文件所属权限
1 2
| hadoop fs -chmod 666 /test/1.txt hadoop fs -chown hadoop:hadoop /test/1.txt
|
-cp
:从 HDFS 的一个路径拷贝到 HDFS 的另一个路径
1
| hadoop fs -cp /test1/1.txt /test2
|
1
| hadoop fs -mv /test1/1.txt /test2
|
1
| hadoop fs -tail /test/1.txt
|
1
| hadoop fs -rm /test/1.txt
|
1 2 3 4 5 6
| [hadoop@hadoop1 hadoop-3.3.1]$ hadoop fs -du -s -h /input/1.txt 37 111 /input/1.txt [hadoop@hadoop1 hadoop-3.3.1]$ hadoop fs -du -h /input 37 111 /input/1.txt 5 15 /input/2.txt # 37表示文件大小;111表示37*3个副本;/input表示查看的目录
|
1 2
| hadoop fs -setrep 10 /input/1.txt # 这里设置的副本数只是记录在NameNode的元数据中,是否真的会有这么多副本,还得看DataNode的数量。因为目前只有3台设备,最多也就3个副本,只有节点数的增加到10台时,副本数才能达到10。
|
5. HDFS的API操作
5.1 HDFS API介绍
涉及的主要类:
- Configuration: 该类的对象封转了客户端或者服务器的配置。
- FileSystem: 该类的对象是一个文件系统对象,可以用该对象的一些方法来对文件进行操作,通过 FileSystem 的静态方法 get 获得该对象。
1
| FileSystem fs = FileSystem.get(conf);
|
- get 方法从 conf 中的一个参数 fs.defaultFS 的配置值判断具体是什么类型的文件系统。如果我们的代码中没有指定 fs.defaultFS,并且工程 classpath 下也没有给定相应的配置,conf 中的默认值就来自于 hadoop 的 jar 包中的 core-default.xml,默认值为:file:///,则获取的将不是一个 DistributedFileSystem 的实例,而是一个本地文件系统的客户端对象。
Java API官方文档:https://hadoop.apache.org/docs/r3.3.1/api/index.html
5.2 环境配置
- 安装包解压在英文路径下
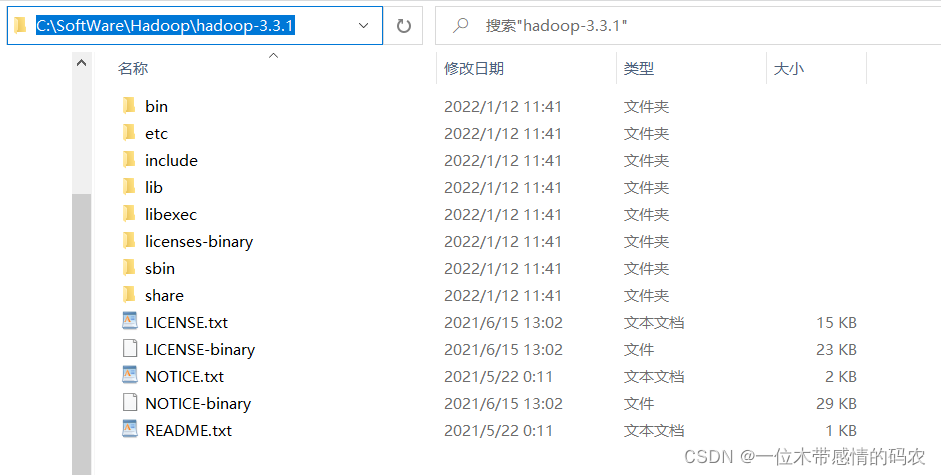
- 配置环境变量
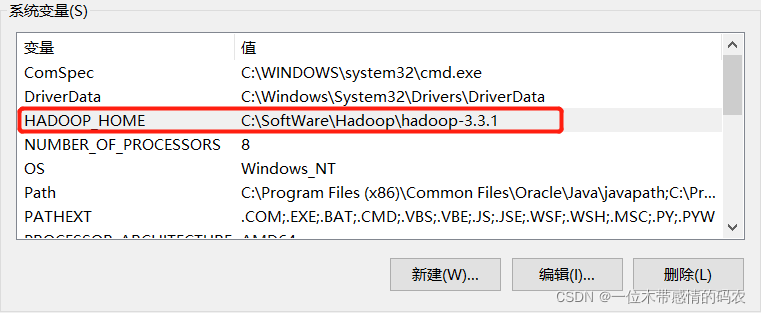
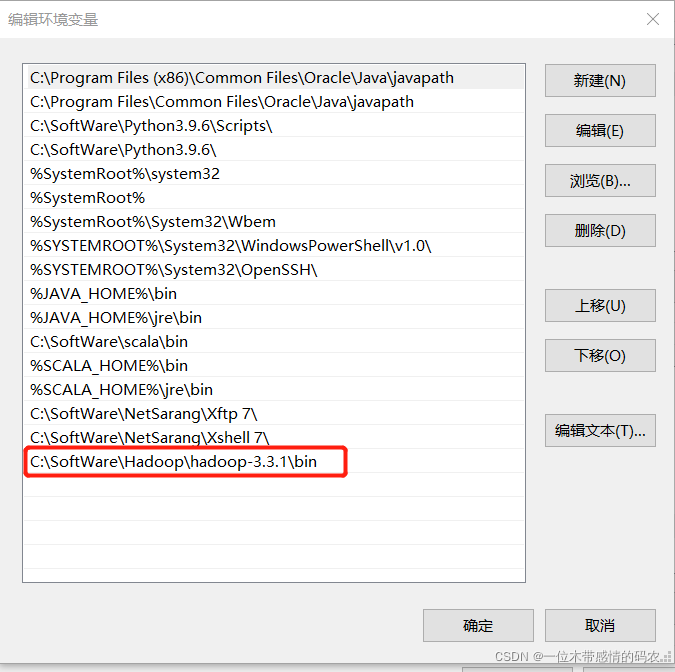
- IDEA 新建Maven项目,加入下面的 pom 依赖
1 2 3 4 5 6 7 8 9 10 11 12 13 14 15 16 17 18 19 20 21 22 23 24 25 26 27 28 29 30 31 32 33 34 35 36 37 38 39 40 41 42 43 44 45 46 47 48 49 50 51 52 53 54 55 56 57 58 59 60 61 62 63 64 65 66 67 68 69 70 71 72 73 74 75 76 77 78 79 80 81 82 83 84 85 86 87 88 89 90 91 92 93 94 95 96 97 98 99 100 101 102 103 104 105 106 107
| <repositories> <repository> <id>cental</id> <url>http://maven.aliyun.com/nexus/content/groups/public//</url> <releases> <enabled>true</enabled> </releases> <snapshots> <enabled>true</enabled> <updatePolicy>always</updatePolicy> <checksumPolicy>fail</checksumPolicy> </snapshots> </repository> </repositories>
<dependencies> <dependency> <groupId>org.apache.hadoop</groupId> <artifactId>hadoop-common</artifactId> <version>3.3.1</version> </dependency> <dependency> <groupId>org.apache.hadoop</groupId> <artifactId>hadoop-client</artifactId> <version>3.3.1</version> </dependency> <dependency> <groupId>org.apache.hadoop</groupId> <artifactId>hadoop-hdfs</artifactId> <version>3.3.1</version> </dependency> <dependency> <groupId>org.apache.hadoop</groupId> <artifactId>hadoop-mapreduce-client-core</artifactId> <version>3.3.1</version> </dependency> <dependency> <groupId>junit</groupId> <artifactId>junit</artifactId> <version>4.13</version> </dependency>
<dependency> <groupId>com.github.pcj</groupId> <artifactId>google-options</artifactId> <version>1.0.0</version> </dependency> <dependency> <groupId>commons-io</groupId> <artifactId>commons-io</artifactId> <version>2.6</version> </dependency> </dependencies>
<build> <plugins> <plugin> <groupId>org.apache.maven.plugins</groupId> <artifactId>maven-compiler-plugin</artifactId> <version>3.1</version> <configuration> <source>1.8</source> <target>1.8</target> </configuration> </plugin>
<plugin> <groupId>org.apache.maven.plugins</groupId> <artifactId>maven-shade-plugin</artifactId> <version>3.1.1</version> <configuration> </configuration> <executions> <execution> <phase>package</phase> <goals> <goal>shade</goal> </goals> <configuration> <createDependencyReducedPom>false</createDependencyReducedPom> <shadedArtifactAttached>true</shadedArtifactAttached> <shadedClassifierName>jar-with-dependencies</shadedClassifierName> <filters> <filter> <artifact>*:*</artifact> <excludes> <exclude>META-INF/*.SF</exclude> <exclude>META-INF/*.DSA</exclude> <exclude>META-INF/*.RSA</exclude> </excludes> </filter> </filters> <transformers> <transformer implementation="org.apache.maven.plugins.shade.resource.ServicesResourceTransformer"/> <transformer implementation="org.apache.maven.plugins.shade.resource.ManifestResourceTransformer"> <mainClass>cn.itcast.sentiment_upload.Entrance</mainClass> </transformer> </transformers> </configuration> </execution> </executions> </plugin> </plugins> </build>
|
- 在
resources
下新建log4j.properties
,加入下面内容
1 2 3 4 5 6 7 8
| log4j.rootLogger=INFO, stdout log4j.appender.stdout=org.apache.log4j.ConsoleAppender log4j.appender.stdout.layout=org.apache.log4j.PatternLayout log4j.appender.stdout.layout.ConversionPattern=%d %p [%c] - %m%n log4j.appender.logfile=org.apache.log4j.FileAppender log4j.appender.logfile.File=target/spring.log log4j.appender.logfile.layout=org.apache.log4j.PatternLayout log4j.appender.logfile.layout.ConversionPattern=%d %p [%c] - %m%n
|
- 再按照Hadoop3.x报错[main] DEBUG [org.apache.hadoop.util.Shell] - Failed to find winutils.exe该博客教程上传文件即可。
5.3 HDFS创建目录
1 2 3 4 5 6 7 8 9 10 11
| @Test public void testMkdirs() throws IOException, URISyntaxException, InterruptedException {
Configuration configuration = new Configuration(); FileSystem fs = FileSystem.get(new URI("hdfs://192.168.68.101:8020"), configuration,"hadoop"); fs.mkdirs(new Path("/hdfsapi")); fs.close(); }
|
5.4 HDFS文件上传
1 2 3 4 5 6 7 8 9 10 11 12 13 14
| @Test public void testCopyFromLocalFile() throws IOException, InterruptedException, URISyntaxException {
Configuration configuration = new Configuration(); configuration.set("dfs.replication", "2"); FileSystem fs = FileSystem.get(new URI("hdfs://192.168.68.101:8020"), configuration, "hadoop");
fs.copyFromLocalFile(new Path("D:/test.txt"), new Path("/input"));
fs.close(); }
|
5.5 HDFS文件下载
1 2 3 4 5 6 7 8 9 10 11 12 13 14 15 16 17
| @Test public void testCopyToLocalFile() throws IOException, InterruptedException, URISyntaxException{
Configuration configuration = new Configuration(); FileSystem fs = FileSystem.get(new URI("hdfs://192.168.68.101:8020"), configuration, "hadoop");
fs.copyToLocalFile(false, new Path("/input/1.txt"), new Path("D:/1.txt"), true);
fs.close(); }
|
5.6 HDFS文件更名和移动
1 2 3 4 5 6 7 8 9 10 11 12 13
| @Test public void testRename() throws IOException, InterruptedException, URISyntaxException{
Configuration configuration = new Configuration(); FileSystem fs = FileSystem.get(new URI("hdfs://192.168.68.101:8020"), configuration, "hadoop");
fs.rename(new Path("/input/test.txt"), new Path("/input/test1.txt"));
fs.close(); }
|
5.7 HDFS删除文件和目录
1 2 3 4 5 6 7 8 9 10 11 12 13
| @Test public void testDelete() throws IOException, InterruptedException, URISyntaxException{
Configuration configuration = new Configuration(); FileSystem fs = FileSystem.get(new URI("hdfs://192.168.68.101:8020"), configuration, "hadoop");
fs.delete(new Path("/output"), true);
fs.close(); }
|
5.8 HDFS文件详情查看
查看文件名称、权限、长度、块信息。
1 2 3 4 5 6 7 8 9 10 11 12 13 14 15 16 17 18 19 20 21 22 23 24 25 26 27 28 29 30
| @Test public void testListFiles() throws IOException, InterruptedException, URISyntaxException {
Configuration configuration = new Configuration(); FileSystem fs = FileSystem.get(new URI("hdfs://192.168.68.101:8020"), configuration, "hadoop");
RemoteIterator<LocatedFileStatus> listFiles = fs.listFiles(new Path("/"), true);
while (listFiles.hasNext()) { LocatedFileStatus fileStatus = listFiles.next();
System.out.println("========" + fileStatus.getPath() + "========="); System.out.println(fileStatus.getPermission()); System.out.println(fileStatus.getOwner()); System.out.println(fileStatus.getGroup()); System.out.println(fileStatus.getLen()); System.out.println(fileStatus.getModificationTime()); System.out.println(fileStatus.getReplication()); System.out.println(fileStatus.getBlockSize()); System.out.println(fileStatus.getPath().getName());
BlockLocation[] blockLocations = fileStatus.getBlockLocations(); System.out.println(Arrays.toString(blockLocations)); } fs.close(); }
|
5.9 HDFS文件和文件夹判断
1 2 3 4 5 6 7 8 9 10 11 12 13 14 15 16 17 18 19 20 21 22 23
| @Test public void testListStatus() throws IOException, InterruptedException, URISyntaxException{
Configuration configuration = new Configuration(); FileSystem fs = FileSystem.get(new URI("hdfs://192.168.68.101:8020"), configuration, "hadoop");
FileStatus[] listStatus = fs.listStatus(new Path("/input"));
for (FileStatus fileStatus : listStatus) {
if (fileStatus.isFile()) { System.out.println("f:"+fileStatus.getPath().getName()); }else { System.out.println("d:"+fileStatus.getPath().getName()); } }
fs.close(); }
|